
How AI Can Reduce Equity Research Costs by 40%: Strategies & Historical Insights
| 7 min read | by James YerkessIn today's rapidly evolving financial landscape, equity research remains essential for informed investment decisions. However, the substantial costs of conducting thorough research can present a significant challenge. Artificial intelligence (AI) has recently emerged as a transformative force, offering the potential to reduce equity research costs by up to 40%.
This article delves into the historical evolution of equity research. It explores how AI can streamline research processes, providing actionable strategies for maintaining quality while cutting costs.
The Evolution of Equity Research: A Historical Overview
Equity research has undergone several key transformations driven by technological innovation over the decades. These advancements have consistently enhanced the capabilities of financial analysts, leading to significant changes in how research is conducted.
-
The Spreadsheet Revolution (1970s-1980s): The introduction of tools such as Lotus 1-2-3, followed by Microsoft Excel, revolutionised financial analysis. These tools enabled analysts to perform more sophisticated calculations and data modelling efficiently.
-
The Rise of Bloomberg Terminals (Mid-1980s): The proliferation of Bloomberg terminals, combined with the growing accessibility of the Internet, transformed the dissemination and consumption of financial information, making real-time data analysis possible.
-
Regulation (2000s): The 2000s saw significant regulatory shifts, including the implementation of MiFID II in Europe and Reg FD in the U.S. These regulations redefined the relationship between buy-side and sell-side analysts, pushing for greater transparency and altering the flow of information.
-
The Age of Alternative Date (from the 2010s): The 2010s introduced alternative data sources and expert networks, providing analysts with new avenues for generating and validating investment ideas, further expanding the scope of equity research.
-
The AI Revolution (from 2024): Today, AI and machine learning are poised to revolutionise equity research. The two key pillars of AI in equity research are structuring unstructured data and enhanced automation of routine tasks.
The Role of AI in Reducing Equity Research Costs
A typical buy-side equity analyst spends about 60 hours a week on various tasks. Some of the tasks are communication tasks: speaking with management, attending industry conferences, speaking with the sell-side or with peers. At least in its current state, AI is not able to automate these tasks.
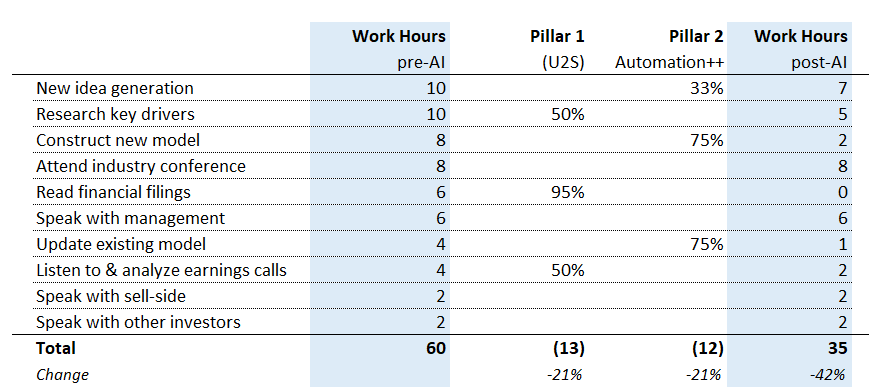
However, AI has the potential to significantly reduce the time and resources required for equity research by automating and enhancing several key processes. AI can make a substantial difference in two key pillars: Structuring Unstructured Data and Enhanced Automation of Routine Tasks.
Pillar 1: Structuring Unstructured Data (U2S)
One of AI's most impactful contributions to equity research is its ability to process and structure vast amounts of unstructured data. We abbreviate this as U2S or Unstructured to Structured.
Tasks in this pillar include:
- Parsing Financial Filings: AI tools can swiftly extract and summarise crucial information from complex financial documents, reducing the need for manual reading and analysis.
- Earnings Calls Analysis: AI can analyse earnings calls, identify key insights, and cut the time analysts spend on these calls by up to 50%. A good example is the analysis completed on the latest Nvidia earnings report by the MarvinLabs App .
- Researching Key Drivers: AI can streamline the process of identifying and analysing the primary drivers behind a company's performance, halving the required time.
Pillar 2: Enhanced Automation of Routine Tasks (Automation++)
The second pillar where AI can reduce costs is through the enhanced automation of routine tasks. We abbreviate this as _Automation++_as it is a continuation of the automation trends in the industry.
A typical equity research analyst dedicates many hours each week to various tasks. AI can significantly reduce the time spent on several of these activities:
- New Idea Generation: AI can assist in generating investment ideas by rapidly scanning large datasets to identify potential opportunities.
- Updating and Creating Models: AI-driven tools can automate updating financial models based on new data, freeing analysts to focus on higher-level analysis.
Reducing Overall Workload by 40%
AI can reduce the overall workload of an equity research analyst by up to 40%. This reduction not only lowers costs but also allows analysts to devote more time to strategic decision-making and in-depth analysis, thereby increasing the overall value of the research process.
Morgan Stanley Wealth Management (MSWM) recently announced the next innovation milestone in its AI @ Morgan Stanley suite of GenAI tools for Financial Advisors to save time and cut costs. The new AI @ Morgan Stanley Debrief is an OpenAI-powered tool that, with client consent, generates notes on a Financial advisor's behalf in client meetings and surfaces action items. The goal is to use AI to reduce workload and costs.
Strategies to Maximise Cost Savings in Equity Research
Incorporating AI into your equity research process is just one piece of the puzzle. To fully capitalise on cost-saving opportunities, consider the following strategies:
-
Outsourcing Research Tasks: Outsourcing routine tasks, such as data collection and preliminary analysis, to specialised firms can further reduce costs. This approach allows in-house analysts to focus on more complex, value-added activities.
-
Leveraging Open-Source and Proprietary Data: While proprietary data sources are essential, integrating open-source data can supplement your analysis at no extra cost. AI can help merge these datasets effectively, providing comprehensive insights.
-
Streamlining Research Processes: AI tools can identify inefficiencies in your current research processes, helping you streamline workflows and reduce redundancy. This leads to faster turnaround times and lower operational costs.
-
Collaborative Research Initiatives: Collaborating with other institutions on research projects can spread the costs and provide access to a broader range of data and expertise, enhancing the overall quality of your research while keeping expenses in check.
Bringing It All Together
The integration of AI into equity research represents the latest chapter in the field's ongoing evolution. AI offers the potential to reduce research costs by up to 40%.
When combined with strategies like outsourcing, leveraging open-source data, and streamlining workflows, AI apps can help financial institutions maintain high-quality research while significantly cutting costs. Embracing these innovations will make equity research more cost-effective and insightful, driving better investment decisions in an increasingly competitive market.
Frequently Asked Questions about AI in Equity Research
Q1: What impact does AI have on equity research?
AI significantly reduces the time and cost associated with various research tasks, particularly those involving unstructured data.
Q2: How can AI reduce equity research costs?
AI automates time-consuming tasks such as data analysis, model updates, and earnings call reviews, potentially reducing overall research costs by up to 40%.
Q3: Is AI replacing human analysts in equity research?
No, AI is not replacing analysts but rather enhancing their capabilities by taking over routine tasks, allowing them to focus on more strategic analysis.
Q4: What are the challenges of integrating AI into equity research?
Challenges include the initial cost of AI tools, the need for training, and ensuring that AI-generated insights meet quality standards.
Q5: How has equity research evolved over the decades?
Equity research has evolved through several technological waves, from the introduction of spreadsheets in the 1970s to the current AI revolution.
Q6: What future trends can we expect in equity research?
Future trends include greater integration of AI, increased reliance on alternative data sources, and more collaborative research initiatives across institutions.